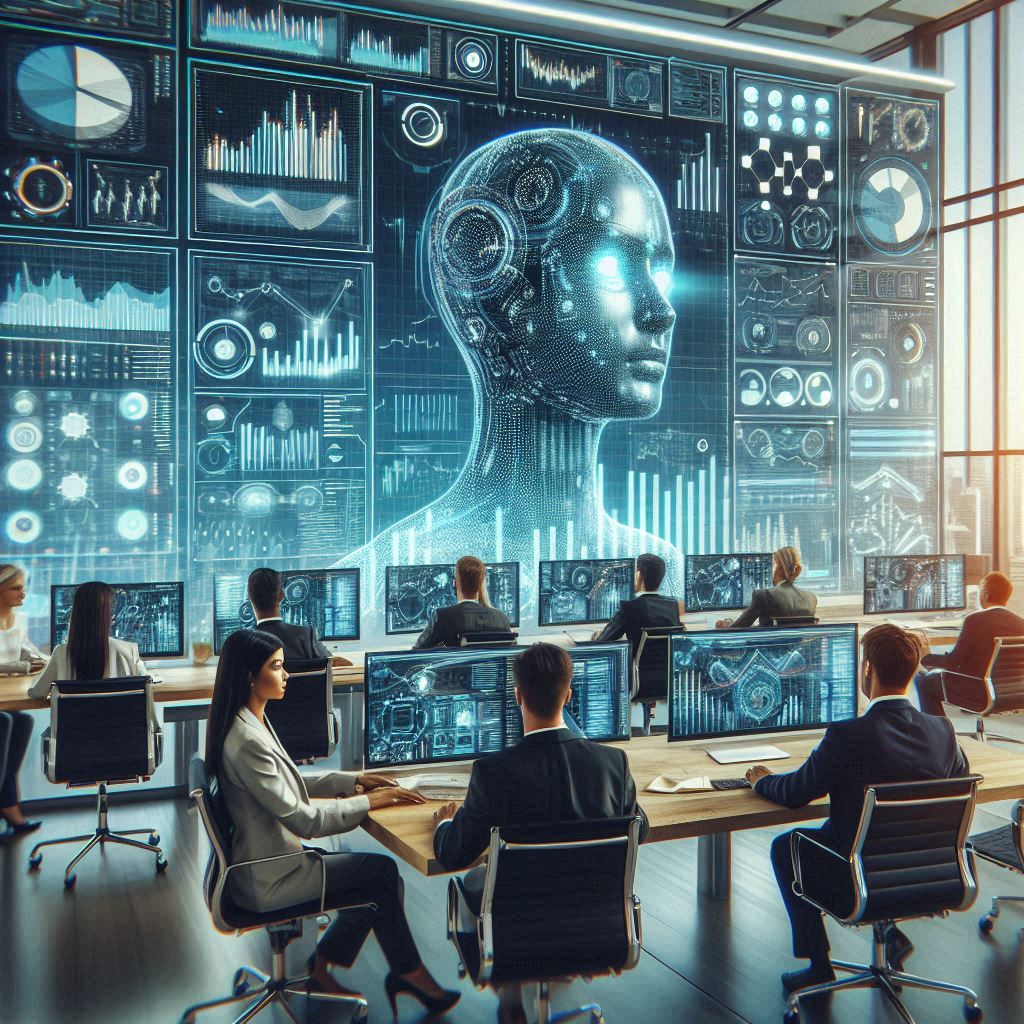
AI is significantly reshaping finance and stock trading by providing tools for enhanced analysis, automation, and decision-making through technologies like machine learning and natural language processing.
Our podcast explores various applications of AI agents, including algorithmic trading, risk management, fraud detection, and portfolio optimization, and highlights prominent AI platforms available to different users.
We also discusses the role of managed service providers in AI implementation, the advantages AI offers such as improved prediction and efficiency, and the challenges and risks associated with its adoption, like bias and regulatory hurdles.
Finally, we presents real-world case studies of successful AI integration and outlines future trends in this rapidly evolving field.
Please listen our podcast about AI in Financial and Stock Trading
Frequently Asked Questions: AI in Financial Trading
1. How is artificial intelligence (AI) currently transforming the financial and stock trading industries?
AI is profoundly reshaping finance and stock trading by providing technologies that emulate human intelligence for analyzing, managing, investing, and protecting financial resources. This includes machine learning (ML), deep learning (DL), natural language processing (NLP), and predictive analytics. These tools enable financial institutions to extract deeper insights from massive datasets, automate complex processes, and make more informed and efficient decisions across areas like algorithmic trading, risk management, fraud detection, and portfolio optimization.
2. What are some key applications of AI agents in financial and stock trading?
AI agents are being applied across a wide range of financial activities. In algorithmic trading, AI executes trades based on pre-programmed rules and data analysis, enabling high-frequency trading. For risk management, AI analyzes large datasets to identify patterns and assess creditworthiness, proactively mitigating market, credit, and operational risks, including cyber threats. In fraud detection, AI identifies unusual transaction patterns. For portfolio optimization, AI selects assets aligning with investor goals and risk tolerance, dynamically adjusting allocations based on market analysis.
3. Can you provide examples of AI-powered platforms and their specific functionalities in financial trading?
Several AI-powered platforms cater to various user needs. Trade Ideas offers real-time market scanning and AI-generated trading signals. TrendSpider focuses on no-code AI strategy creation and automated technical analysis. Intellectia provides in-depth stock analysis and an AI stock picker. Tickeron offers AI trend forecasting and automated trading robots. Stock Hero allows easy bot setup and access to a strategy marketplace. QuantConnect is a platform for developing and deploying algorithmic trading strategies. These platforms highlight the diversity of AI applications, from generating trading signals to automating entire trading strategies.
4. What are the main advantages of implementing AI in financial and stock trading operations?
Implementing AI offers numerous benefits, including enhanced prediction accuracy and speed in analyzing vast datasets, leading to more informed trading decisions. AI provides advanced risk management through sophisticated fraud detection and proactive risk mitigation. It also offers cost efficiency by automating routine tasks and reducing analysis time. Furthermore, AI enables backtesting and strategy optimization, real-time market sentiment analysis, personalization of trading strategies, improved regulatory compliance, and 24/7 operation without emotional biases.
5. What are the significant risks and challenges associated with the adoption of AI in financial trading?
Despite the advantages, AI implementation presents challenges. Biases in algorithms from skewed data can lead to misleading analyses and discriminatory outcomes. Regulatory challenges exist due to the need for frameworks governing AI use in areas like market manipulation and data privacy. The complexity and “black box” nature of some AI models can hinder auditing and risk management. AI’s adaptability to unfamiliar market conditions and potential over-reliance on historical data are concerns. Systemic risks from widespread use of similar AI strategies could increase market instability. Finally, data privacy and security and the need for human oversight remain critical considerations.
6. How are Managed Services Providers (MSPs) assisting financial institutions with AI adoption?
MSPs play a vital role in facilitating AI adoption by helping financial institutions assess their AI readiness, develop AI roadmaps, and implement tailored solutions. They offer expertise in optimizing data management, ensuring data quality and governance. MSPs also assist in customizing AI models and integrating user-friendly interfaces. Partnering with MSPs provides cost-effective access to specialized expertise, ensures robust and secure AI implementations compliant with regulations, improves data quality and speed, enhances customer service through AI-powered support, and offers ongoing support and maintenance.
7. Can you provide examples of real-world successful integrations of AI in the financial sector?
Several financial institutions have successfully integrated AI. Quantitative hedge funds like Renaissance Technologies and Two Sigma have achieved substantial returns using AI models. BlackRock’s Aladdin platform uses AI for risk assessment and portfolio optimization. FinSecure Bank reduced fraud by 60% with an AI-driven system. QuickLoan Financial improved its loan approval process with AI. CapitalGains Investments saw a 20% increase in annual returns by integrating AI into its investment strategy. JPMorgan Chase uses AI chatbots for customer service and the COiN platform to review legal documents efficiently.
8. What are some anticipated future trends and advancements in AI for financial and stock trading?
The future of AI in financial trading is expected to see increased use of deep learning for more accurate predictions and the rise of AI-powered hedge funds. Integration with technologies like blockchain for enhanced security is anticipated. NLP and sentiment analysis will likely play a larger role in extracting insights from unstructured data. Predictive analytics will further refine market forecasting. “Quantamental investing,” combining quantitative and fundamental analysis with AI, is expected to grow. The emergence of agent-based AI could lead to more autonomous financial operations.
Related Posts
Healthcare Data Archiving
Cloud-Based Healthcare Data Archiving Solutions Healthcare data archiving solutions are specialized systems crucial for securely storing…
Digital Infrastructure Strategy
How to Implement a Successful Digital Infrastructure Strategy In today’s hyper-connected world, a robust and…
Boosting Productivity and Efficiency
Boosting Productivity and Efficiency with Intelligent Workplace In today’s fast-evolving business landscape, organizations must rethink…
Model Context Protocol MCP
Understanding the Model Context Protocol (MCP) The Model Context Protocol (MCP) is a new open…